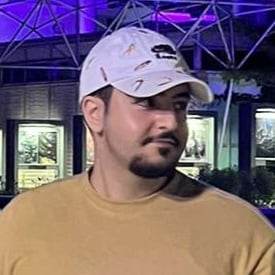
The Fascinating Journey of AI: From Turing to ChatGPT
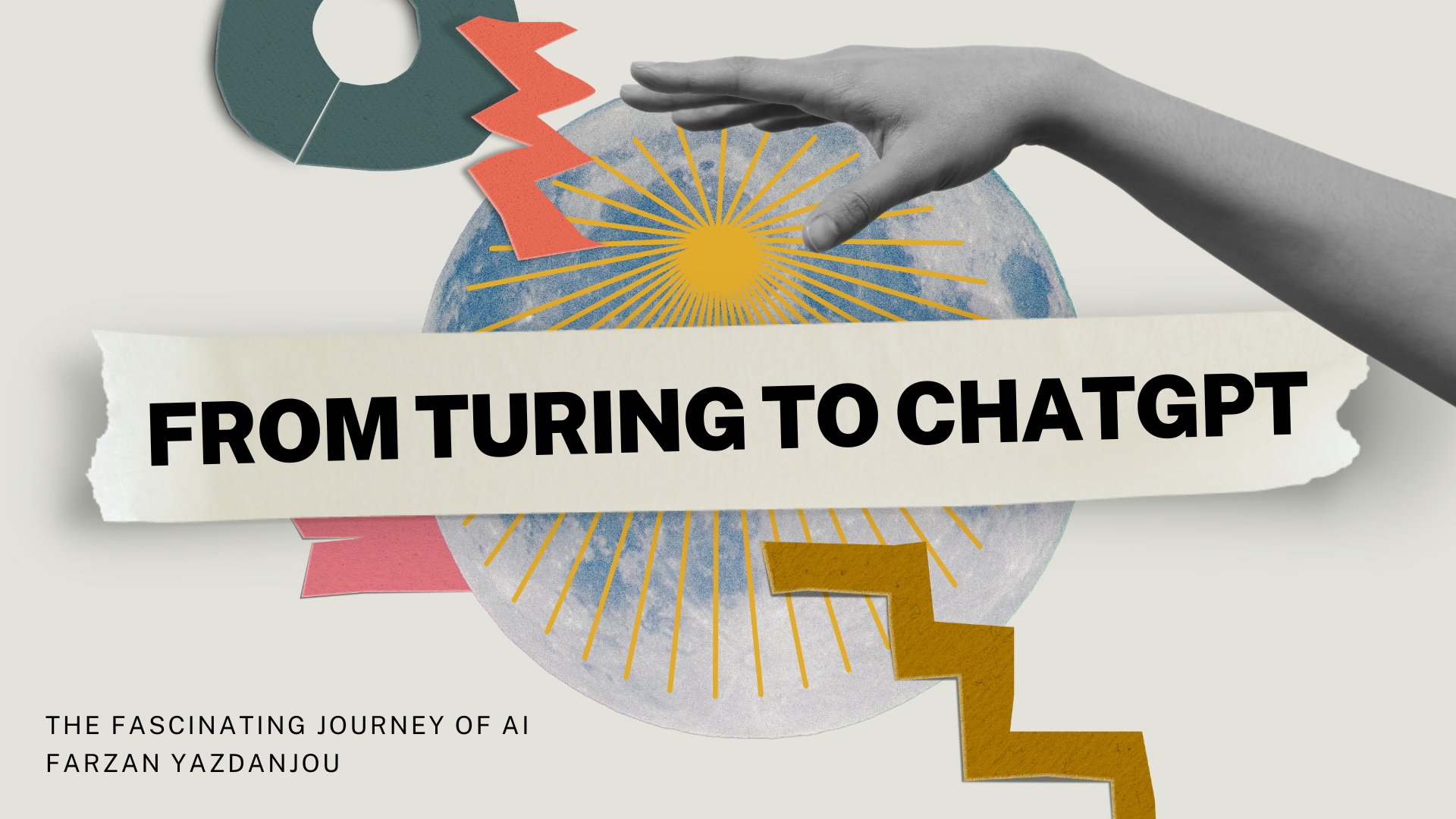
In this post, we embark on an exciting journey through the fascinating history of artificial intelligence, from its early conception with Alan Turing's revolutionary ideas to the awe-inspiring capabilities of ChatGPT.
Introduction
For most people, AI has been a sudden emergence - from vaguely knowing what it is, to hearing about it daily. Even though it feels like it, such impressive technology couldn't have just happened overnight - so where did it all start and how did we get here?
Chapter 1: The Spark That Ignited the Flame
Our journey begins in 1950 when the brilliant mathematician and computer scientist, Alan Turing, proposed a thought experiment that would lay the foundation for the field of artificial intelligence. Turing introduced the concept of a machine capable of mimicking human intelligence so convincingly that it would be indistinguishable from a human in a blind test. This idea became known as the Turing Test and sparked a global pursuit to develop machines that could emulate human cognition.
The Turing Test ignited curiosity and challenged the scientific community to explore the possibility of creating intelligent machines. Over the following decades, researchers from various disciplines delved into the study of logic, problem-solving, learning, and language understanding, all with the goal of developing artificial intelligence. These early efforts paved the way for groundbreaking discoveries and advancements that would shape the landscape of AI as we know it today.
Chapter 2: The Birth of AI as a Field
Fast forward to 1956, when a group of visionaries gathered at the Dartmouth Conference for 8 weeks to discuss the idea of machines that could simulate human intelligence. This historic event marked the birth of AI as a formal field of study and spawned the creation of research labs dedicated to unlocking the mysteries of machine intelligence.
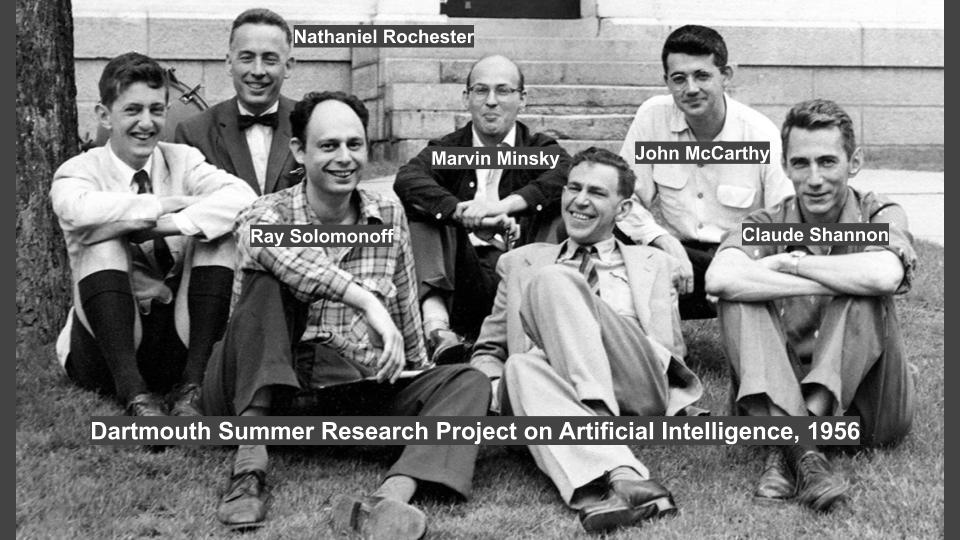
During this period, researchers developed some of the first AI programs, which demonstrated the potential for machines to perform tasks that required human-like intelligence. My favourite example is Arthur Samuel's checkers program, which showcased the ability of a machine to learn from experience.
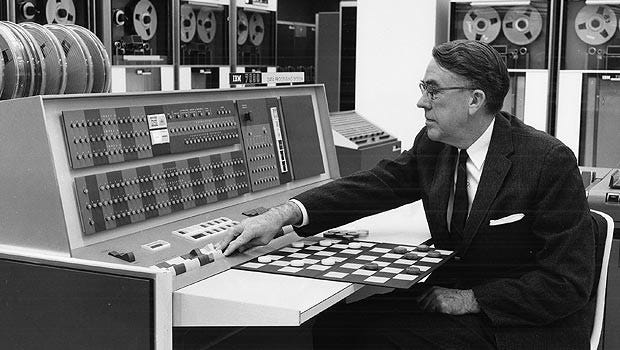
Chapter 3: The AI Winter
The 1970s and 1980s were challenging times for the field of artificial intelligence. Despite early enthusiasm and some impressive achievements, the limitations of AI technology began to surface. Researchers faced significant obstacles in scaling AI systems to handle real-world complexity, and the computational power at the time was inadequate to support more advanced AI models. This period, known as the AI Winter, saw a decline in funding, interest, and progress within the AI research community.
However, the AI Winter was not without its breakthroughs. Researchers continued to explore alternative approaches to AI, leading to the development of expert systems, which were designed to mimic the decision-making process of human experts in specific domains. Additionally, the emergence of new algorithms, such as the Backpropagation Algorithm for training neural networks, laid the groundwork for future advancements in machine learning and deep learning. While the AI Winter dampened expectations, it also set the stage for the resurgence of AI research that would follow in the 1990s and beyond.
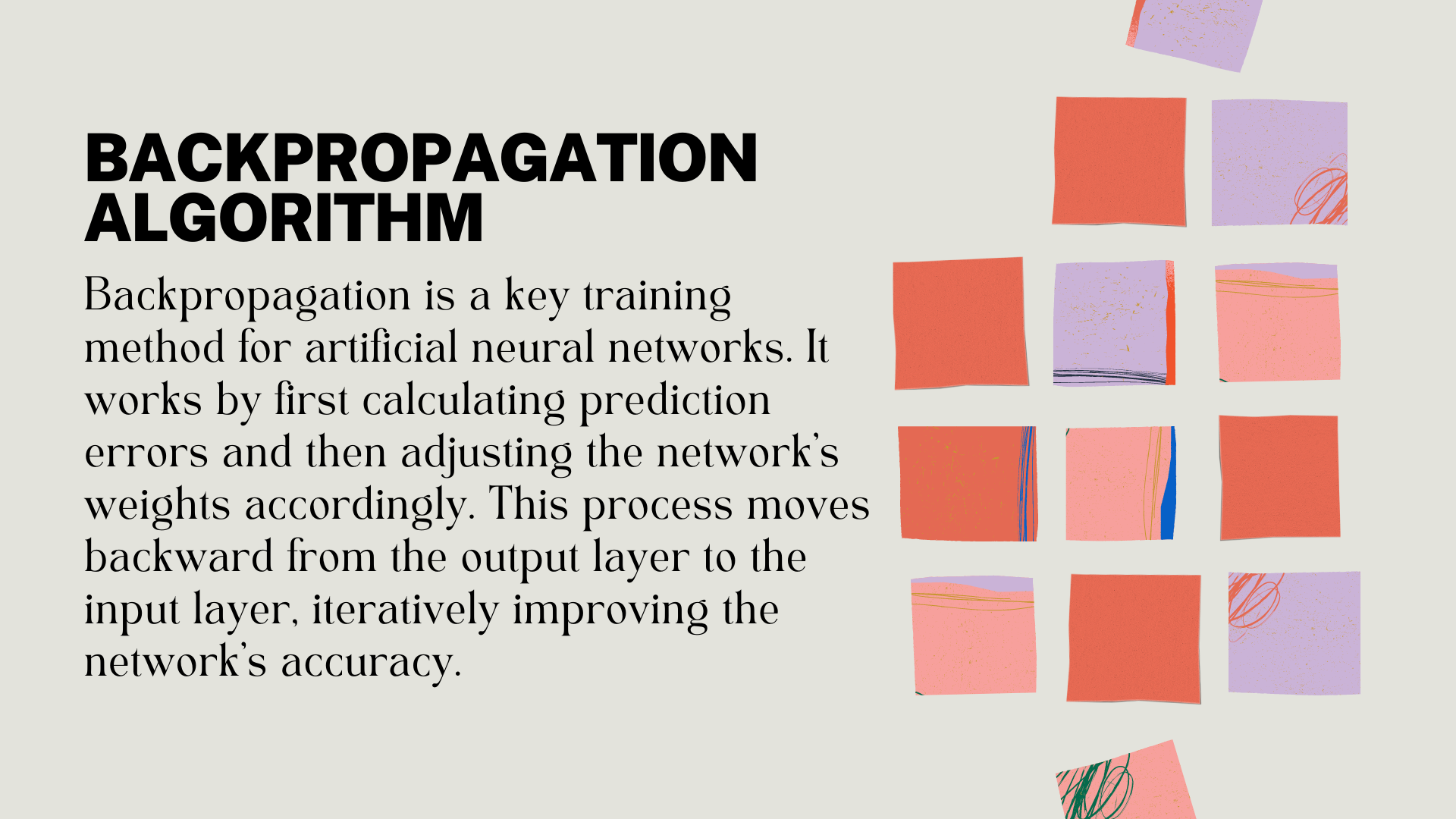
Chapter 4: The Resurgence of AI
In the late 1980s and 1990s, the AI research community experienced a resurgence, driven primarily by the rise of machine learning. Machine learning is a subfield of AI that focuses on creating algorithms capable of learning patterns and making predictions based on data. This shift in approach, from rule-based systems to data-driven models, marked a turning point in the development of AI technology.
During this period, researchers made significant progress in refining machine learning algorithms and techniques. Some notable advancements include the development of Support Vector Machines (SVMs), which are highly effective at classification tasks, and the popularization of ensemble learning methods, such as Random Forests and AdaBoost. These innovations breathed new life into the field of AI, setting the stage for further advancements in the coming years.
Chapter 5: The Rise of Machine Learning Applications
As we entered the 2000s, the AI field continued to gain momentum, building on the progress made in machine learning during the 1990s. This era saw the increasing integration of machine learning models into practical applications, such as search engines, recommendation systems, and spam filters. These applications demonstrated the potential for AI to transform industries and improve our everyday lives.
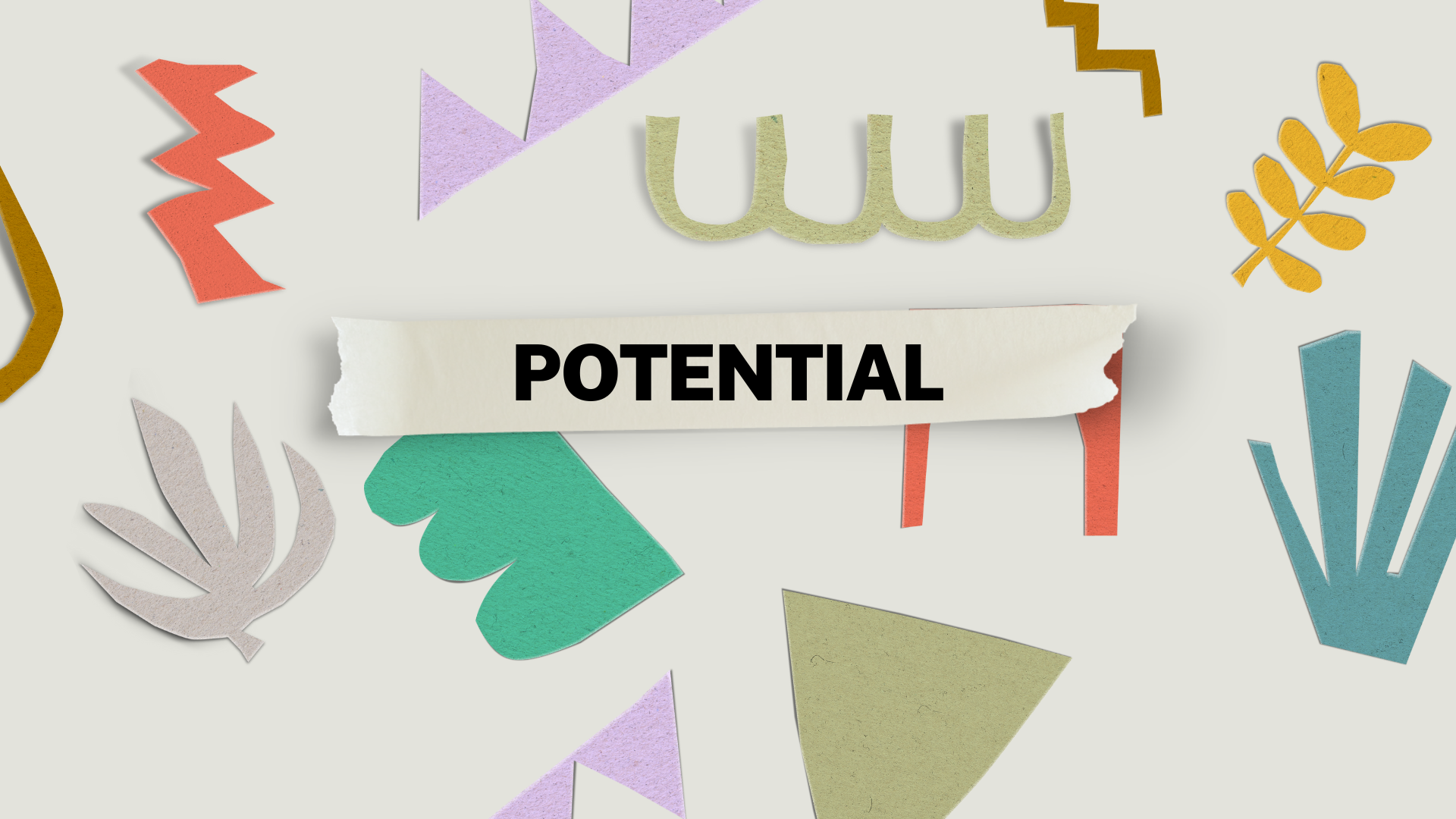
Meanwhile, advancements in machine learning techniques continued at a rapid pace. The development of algorithms like deep learning, reinforcement learning, and unsupervised learning opened new doors for AI research and applications. These methods allowed for more complex pattern recognition and decision-making, further expanding the potential of AI in various fields.
Chapter 6: Big Data: The Fuel for AI's Growth
The 2000s and 2010s witnessed the emergence of big data, which played a crucial role in AI's growth. The explosion of big data can be attributed to factors like widespread digitalization, the proliferation of internet-connected devices, the rise of social media, and advances in data storage and processing. This wealth of data provided AI researchers with the raw material needed to develop sophisticated machine learning models, while increased computational power from GPUs further accelerated AI research.
The convergence of big data and computational power laid the foundation for significant advancements in AI applications, such as speech recognition and computer vision. This set the stage for the incredible AI revolution we witness today.
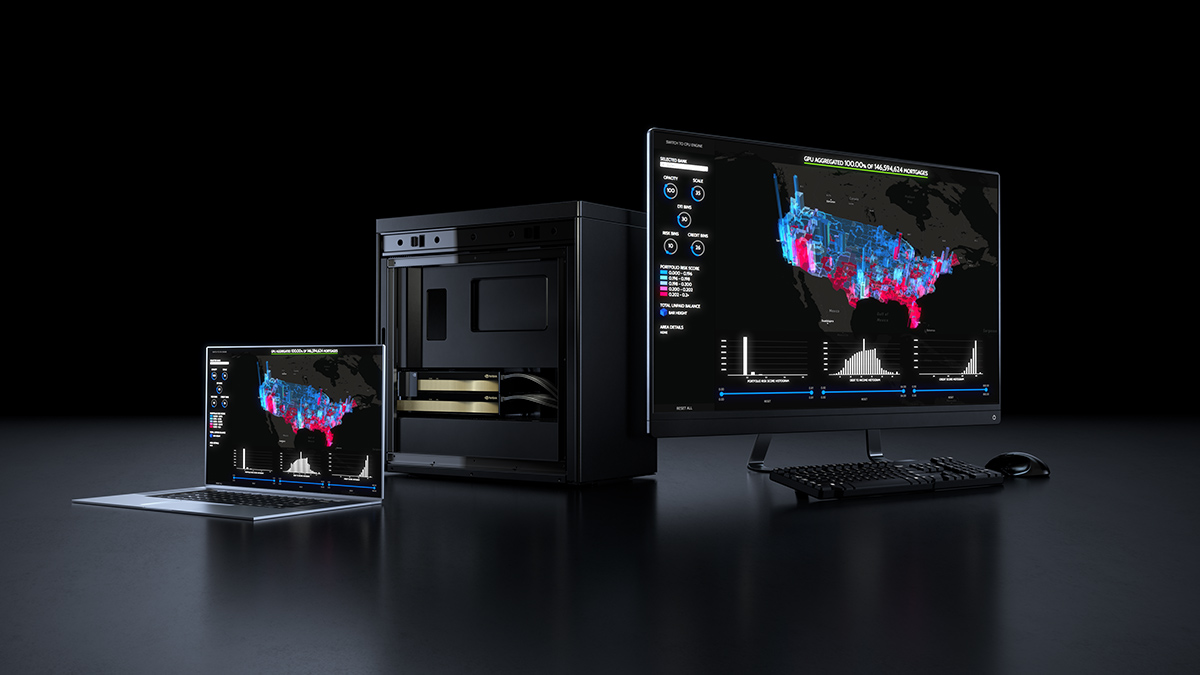
Chapter 7: Unleashing the True Potential of AI
The 2010s marked the rise of deep learning, a subfield of machine learning that unlocked the true potential of AI. Deep learning focuses on the use of artificial neural networks, which consist of multiple layers of interconnected neurons. These networks can learn complex patterns and representations from vast amounts of data, enabling them to excel in tasks that were previously considered challenging for AI. Aided by powerful GPUs and the wealth of big data available, researchers could now train these deep neural networks to achieve unprecedented levels of accuracy and performance.
Key breakthroughs during this period include AlexNet, a deep convolutional neural network that achieved groundbreaking results in image recognition, and BERT, a transformer-based model that revolutionized natural language processing. These breakthroughs not only demonstrated the power of deep learning models but also ignited a surge of interest and investment in AI research. As a result, AI applications have become increasingly sophisticated, leading to the rapid advancement and widespread adoption of AI technology that we see today.
Chapter 8: The Dawning of a New AI Era
OpenAI, a leading AI research organization founded in 2015, has been at the forefront of breakthroughs in natural language processing. The original GPT model, released in 2018, marked a significant step forward in the field. However, GPT did not achieve mainstream adoption due to its limited capabilities and versatility compared to its successors. Additionally, at the time of GPT's release, the AI landscape was still growing, and many organizations had not yet recognized the full potential of AI-powered language models.
In 2019, OpenAI introduced GPT-2, which showcased a substantial leap in performance and functionality, garnering increased interest and awareness in natural language processing. Despite its impressive capabilities, GPT-2's mainstream adoption was delayed due to concerns about potential misuse and the need for further development in infrastructure and tools. This period of evolution and growth paved the way for the arrival of GPT-3 and, eventually, GPT-4, which have since been integrated into mainstream technology.
Chapter 9: GPT-3 - A Giant Leap for AI-kind
In 2020, OpenAI took another giant leap with the release of GPT-3, boasting 175 billion parameters. This astonishing model demonstrated unparalleled capabilities and versatility, generating human-like text across various languages, formats, and topics. One of the remarkable applications of GPT-3 is ChatGPT, which revolutionized conversational AI by providing coherent, contextually relevant, and engaging responses in both single-turn tasks and multi-turn conversations.
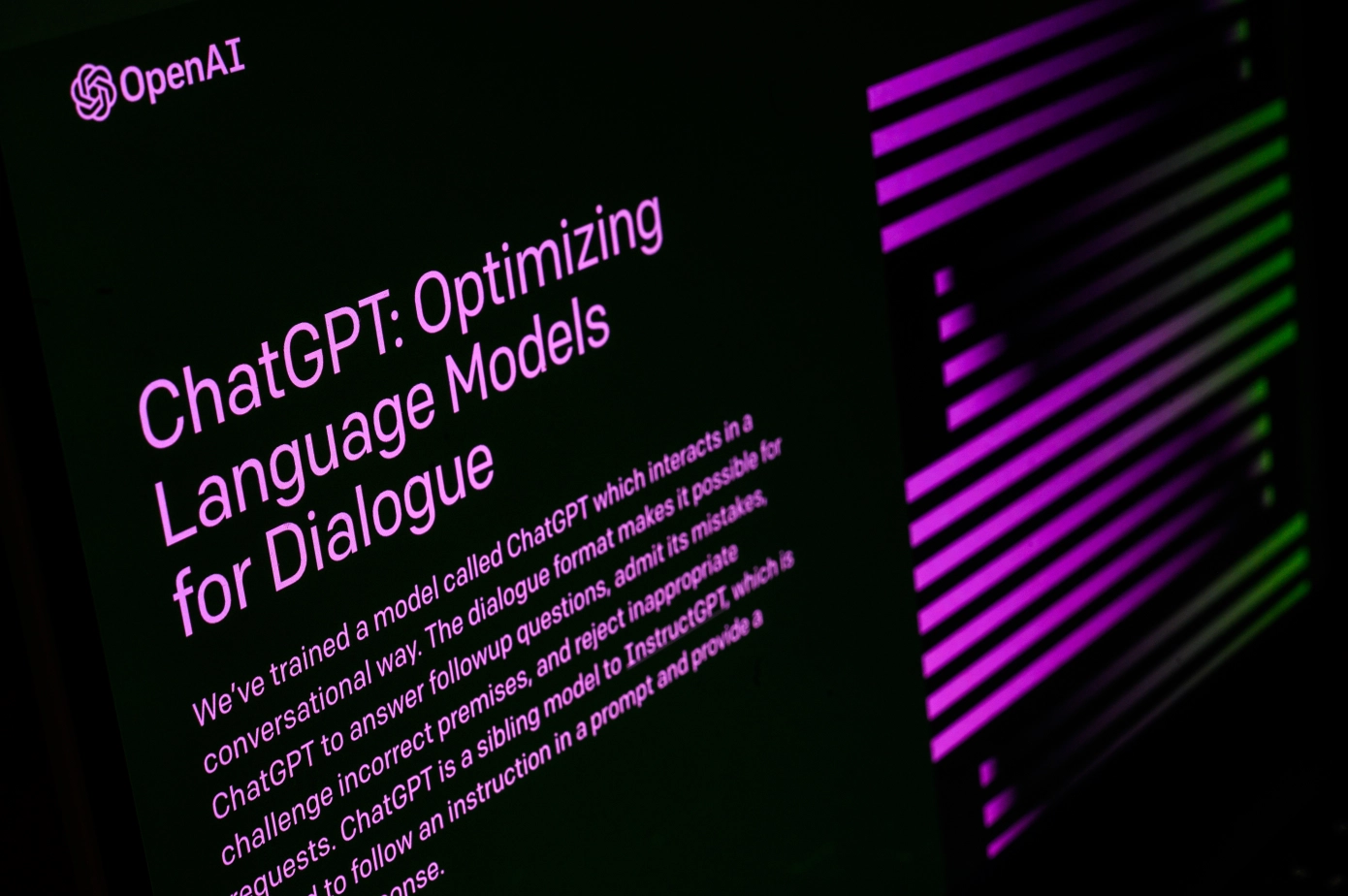
The introduction of ChatGPT marked a turning point in the adoption of AI-powered language models. With 100 million active users just 2 months after its launch in December of 2022, ChatGPT is the fastest-growing consumer application in history. Users around the world rely on ChatGPT for a wide range of applications, including content creation, virtual assistance, customer support, and even programming help. This widespread adoption has been facilitated by OpenAI's API, which enables developers to easily integrate GPT models into their applications and services.
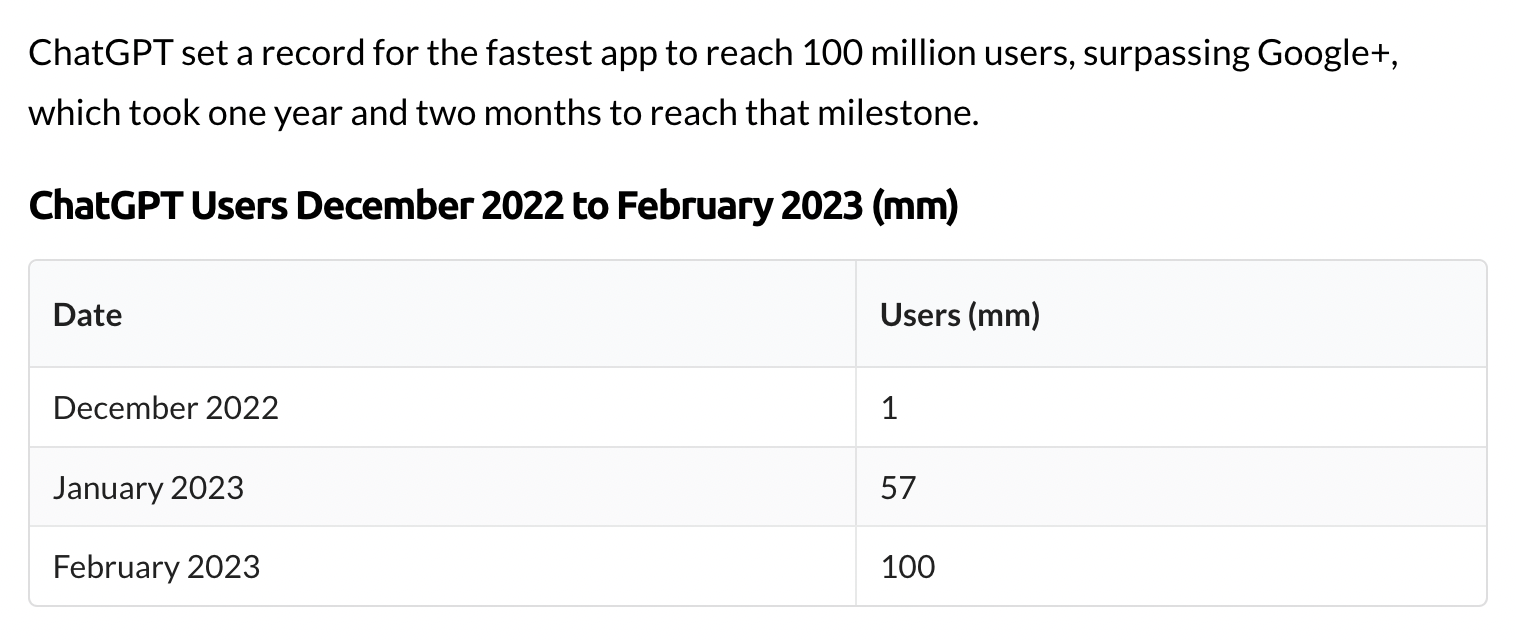
As the number of users and applications of ChatGPT and other GPT-3-based models continues to grow, the tech industry has come to recognize the immense value and potential of AI-powered language models. This momentum has led to the rapid advancement and integration of AI in mainstream technology, culminating in the development and adoption of even more advanced models like GPT-4.
Chapter 10: The Rise of GPT-4 and Mainstream AI Integration
As we arrive in the early 2020s, AI models like GPT-4 have become deeply integrated into mainstream tech products and services. Compared to GPT-3's 175 billion parameters, GPT-4 boasts a tremendous 1.8 trillion - 10 times more. Not only is it smarter, it is also capable of understanding images. If you were to consider the Turing test, GPT-4 would be indistinguishable from the average human in a text-based conversation. The remarkable journey from Turing's original question to the advanced AI systems we see today is a testament to human ingenuity and our unrelenting pursuit of knowledge.
Thank you for reading!
Thank you for joining me on this journey through the world of AI. I hope you enjoyed exploring the incredible milestones that have shaped this fascinating field. Make sure to subscribe for future episodes as we unravel and marvel at the advancements of artificial intelligence.